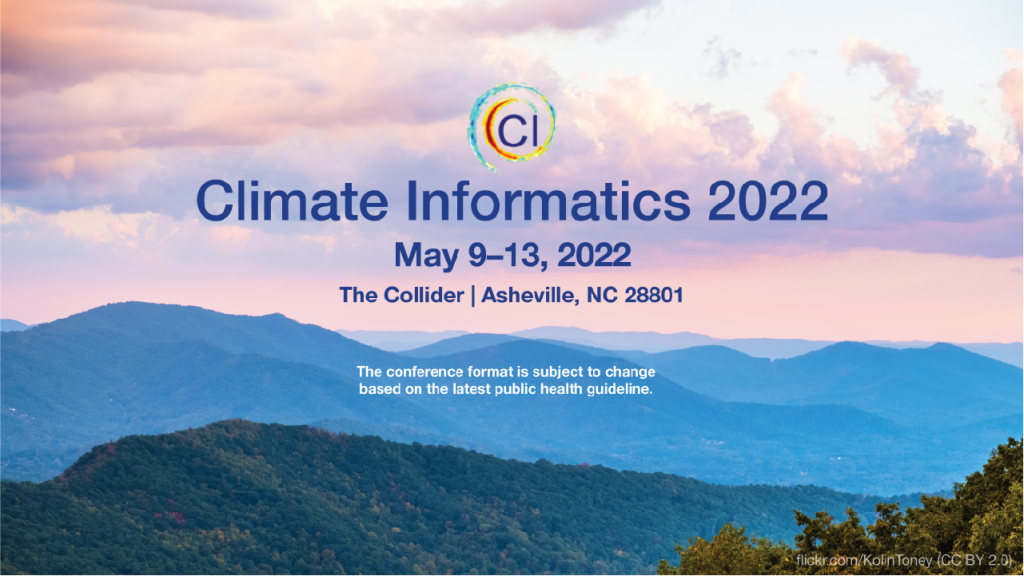
Agenda
- Hackathon (hybrid): 9 May (afternoon) – 10 May (morning)
- Main conference: 10 May (afternoon) – 13 May (morning)
9 May 2022 (Monday)
Time | Talk | Speaker / Format |
---|---|---|
12:00 – 13:00 | Registration | |
13:00 – 17:00 | Hack-a-thon session (hybrid) | Hybrid |
10 May 2022 (Tuesday)
Time | Talk | Speaker / Format |
---|---|---|
09:00 – 12:00 | Registration & Hack-a-thon session (hybrid) | Hybrid |
12:00 – 13:00 | Registration & Lunch break | |
13:00 – 13:10 | Welcome & Conference Logistics | |
13:10 – 14:10 | Data Science and Interdisciplinary Collaboration (Invited) | Alyson Wilson |
14:10 – 14:40 | Coffee break | |
14:40 – 15:00 | Towards data assimilation of ship induced aerosol-cloud interactions | Lekha Patel |
15:00 – 15:20 | Exploring cirrus cloud microphysical properties using explainable machine learning | Kai Jeggle |
15:20 – 15:40 | Stochastic Parameterization of Column Physics using Generative Adversarial Networks | Balasubramanya T Nadiga |
15:40 – 16:00 | Deep prior in variational assimilation to estimate ocean circulation without explicit regularization | Arthur Filoche |
16:00 – 17:00 | Virtual poster session 1 | Virtual |
11 May 2022 (Wednesday)
Time | Talk | Speaker / Format |
---|---|---|
09:00 – 09:10 | Welcome remarks for Day 2 | |
09:10 – 10:10 | Challenges in machine learning for climate extremes (Invited) | Brian White |
10:10 – 10:40 | Coffee break | |
10:40 – 11:00 | ResDeepD: A Residual Super-Resolution Network for Deep Downscaling of Daily Precipitation over India | Sumanta Chandra Mishra Sharma |
11:00 – 11:20 | Week-ahead Solar Irradiance Forecasting with Deep Sequence Learning | Saumya Sinha |
11:20 – 11:40 | SWIFT-AI: Implicit Neural Representations for Stratospheric Ozone Chemistry | Helge Mohn |
11:40 – 12:00 | Lightning: An Essential Climate Variable | Steven J. Goodman |
12:00 – 13:00 | Lunch break | |
13:00 – 13:20 | A Dependent Multi-model Approach to Climate Prediction with Gaussian Processes | Marten Thompson |
13:20 – 13:40 | Physics-Informed Learning of Aerosol Microphysics | Paula Harder |
13:40 – 14:00 | Detection and attribution of climate change: a Deep Learning and Variational approach | Constantin Bône |
14:00 – 14:20 | Learning to construct Probabilistic Multi-Model Ensemble for Seasonal Predictions | Nachiketa Acharya |
14:20 – 15:00 | Coffee break | |
15:00 – 16:00 | Panel Discussion: Cloud Computing in Advancing Climate Informatics | Panelists: Annie Burgess, ESIP; Maureen Taylor, NOAA; Jonathan Brannock, NCICS; Brian White, UNC-Chapel Hill |
16:00 – 17:00 | In-person Poster & Networking Reception | In-person |
12 May 2022 (Thursday)
Time | Talk | Speaker / Format |
---|---|---|
08:30 – 09:30 | Climate-Invariant, Causally Consistent Neural Networks as Robust Emulators of Subgrid Processes across Climates (Invited) | Tom Beucler (Virtual) |
09:30 – 10:00 | Coffee break | |
10:00 – 10:20 | Constructing a graph metadatabase for more effective search and discovery of datasets | Myranda R Uselton |
10:20 – 10:40 | An energy conserving method for simulating heat transfer in permafrost with hybrid modeling | Brian Groenke |
10:40 – 11:00 | A comparison of explainable AI solutions for a climate change prediction task | Philine L Bommer |
11:00 – 11:20 | Modeling Stratospheric Polar Vortex Variation and Identifying Vortex Extremes Using Explainable Neural Networks | Zheng Wu |
11:20 – 12:20 | Virtual poster session 2 | Virtual |
12:20 – 13:25 (revised time) | Lunch break | |
13:25 – 14:25 (revised time) | Asking how the Southern Ocean responds to global heating and understanding why the answer emerged (Invited) | Maike Sonnewald |
14:25 – 14:40 (revised time) | Coffee Break | |
14:40 – 15:00 | Learning the spatio-temporal relationship between wind and significant wave height using deep learning | Said Obakrim |
15:00 – 15:20 | A Gaussian Process State-space Model for Sea Surface Temperature Reconstruction from the Alkenone Paleotemperature Proxy | Taehee Lee |
15:20 – 15:40 | SSH Super-Resolution using high resolution SST with a Subpixel Convolutional Residual Network | Théo Archambault |
15:40 – 16:00 | Forecasting Marine Heatwaves using Machine Learning | Ayush Prasad |
16:00 – 17:00 | Networking session |
13 May 2022 (Friday)
Time | Talk | Speaker |
---|---|---|
09:00 – 10:00 | Virtual Poster Session 3 | Virtual |
10:00 – 10:20 | Coffee break | |
10:20 – 10:40 | Long-term stability and generalization of observationally-constrained stochastic data-driven models for geophysical turbulence | Ashesh K Chattopadhyay |
10:40 – 11:00 | Neural embedding for closure models | Said Ouala |
11:00 – 12:00 | Building Digital Twins of the Earth for NVIDIA’s E-2 Initiative (Invited) | David M. Hall (Virtual) |
12:00 – 12:30 | Closing session | |
12:30 | Attendee depart |