Figure 1: The figure compares front identification results from (a) manually produced data from NOAA’s Coded Surface Bulletin dataset and (b) the DL-FRONT program for 1 August 2009 at 12:00:00.
NCICS researchers Jim Biard and Kenneth Kunkel have developed a deep learning neural network tool called DL-FRONT that automatically identifies weather fronts in datasets of historical weather observations and climate model outputs. By automating a process that normally requires painstaking manual effort, this new tool makes it possible to do science that was previously impracticable. They report on their work in a new paper in Advances in Statistical Climatology, Meteorology and Oceanography.
Weather fronts—cold fronts, warm fronts, stationary fronts, and occluded fronts—help drive the development of severe thunderstorms and other major weather events. In particular, a majority of the extreme precipitation events in the United States are associated with fronts. Forecasters from the National Weather Service (NWS) have been manually identifying weather front locations for many decades, typically by looking at maps generated at 3-hour intervals that show contours of air pressure and numerous point measurements of temperature, humidity, wind speed, and wind direction. However, this is a labor-intensive process: it would take a trained forecaster at least 500 hours to find fronts over North America for a single year’s worth of 3-hour maps. DL-FRONT can perform the same task in minutes, opening up possibilities for new lines of scientific inquiry.
DL-FRONT was trained using five years of information from NOAA’s Coded Surface Bulletin (CSB) dataset—the digital record of the front locations identified by NWS forecasters—paired with maps of pressure, temperature, humidity, and wind from a computer reanalysis model: the NASA Modern-Era Retrospective analysis for Research and Applications, Version 2 (MERRA-2). Reanalysis models like MERRA-2 use large amounts of direct and indirect observations of the atmosphere taken from the ground, from the air, and from space to produce gridded maps of data for times and locations where no direct observations were previously available.
The authors assessed the trained network’s performance by comparing its results against eight years of human-produced weather front locations from the CSB that were set aside for testing. They found that DL-FRONT was around 90% successful in automatically determining the locations of weather fronts over North America when compared against front locations determined manually by forecasters (Figure 1). DL-FRONT’s success rate may be even higher at detecting fronts associated with significant weather events.
The trained model can be used to detect fronts in other observational datasets or in the outputs of climate models. For example, the authors used DL-FRONT to look at the monthly occurrences of fronts over time in both the CSB and MERRA-2 datasets (Figure 2). The results showed good agreement overall but with a small increasing trend in the CSB data that is not reflected in the MERRA-2 reanalysis.
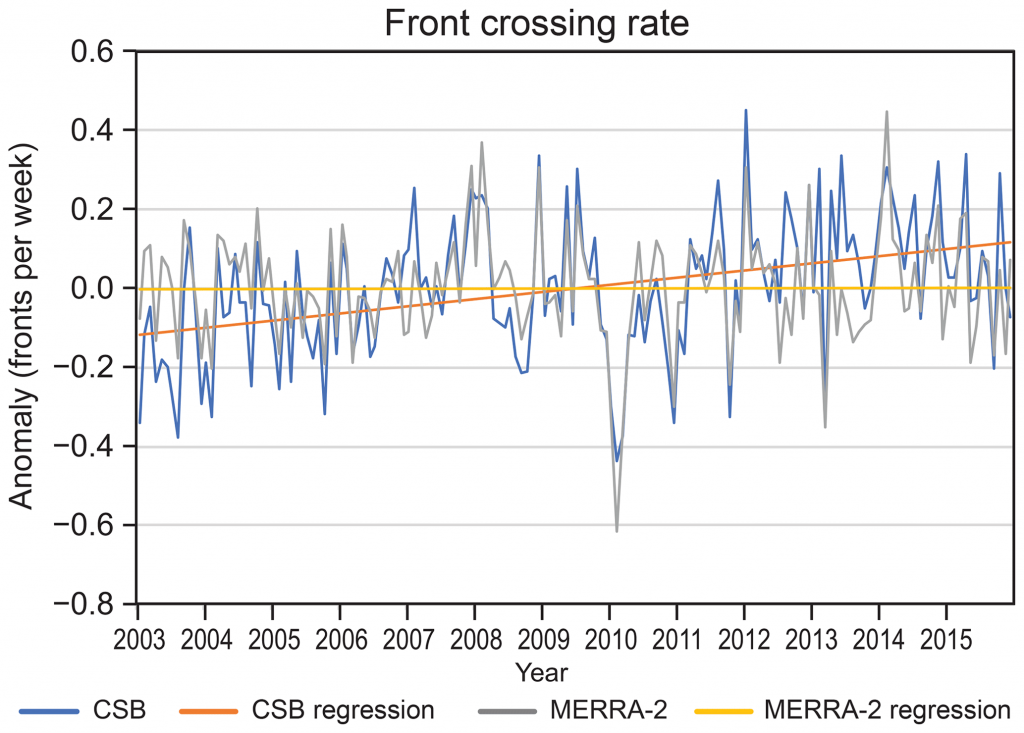
Figure 2: Using DL-FRONT to analyze monthly front crossings in both the Coded Surface Bulletin (CSB) and the MERRA-2 reanalysis datasets shows good agreement between the CSB and MERRA-2 data overall, but it also reveals an upward trend in the number of events in the CSB data that is not present in the MERRA-2 reanalysis.
DL-FRONT can also be used to analyze how the frequency and location of weather fronts may change in the future under different global warming scenarios.
Data and analysis results available on Zenodo via the links below:
- https://doi.org/10.5281/zenodo.2712481 – DL-FRONT MERRA-2 weather front processing and analysis artifacts
- https://doi.org/10.5281/zenodo.2641072 – DL-FRONT MERRA-2 weather front probability maps over North America, 1980–
- https://doi.org/10.5281/zenodo.2669180 – DL-FRONT MERRA-2 vectorized weather fronts over North America, 1980–2018 (JSON format)
- https://doi.org/10.5281/zenodo.2669505 – DL-FRONT MERRA-2 vectorized weather fronts over North America, 1980–2018 (netCDF format)
- https://doi.org/10.5281/zenodo.2642801 – National Weather Service Coded Surface Bulletins, 2003–
- https://doi.org/10.5281/zenodo.2646544 – National Weather Service Coded Surface Bulletins, 2003– (JSON format)
- https://doi.org/10.5281/zenodo.2651361 – National Weather Service Coded Surface Bulletins, 2003– (netCDF format)
Biard, J.C. and K.E. Kunkel, 2019: Automated detection of weather fronts using a deep learning neural network. Advances in Statistical Climatology, Meteorology and Oceanography, 5 (2), 147–160. http://dx.doi.org/10.5194/ascmo-5-147-2019